"How do you give a Data Science bootcamp to a mixed cohort of undergraduate and graduate students from a wide range of disciplines?" This is a question I was facing earlier this year when I needed to design the initiation boot camp for our inaugural class of DSI Scholars. Some of them are rising sophomores, and some of them are PhDs in English. Some of these students had years of coding experience, some of these students had taken a full curriculum in Statistics and Machine Learning, and some of them thought "we probably won't fit in a data science boot camp at all."
The DSI Scholars program is to promote data science research and create a learning community for student researchers, especially during the slow months of summer. The learning goals of the boot camp were designed to be less about specific ML tools or programming languages. Rather it was set out to be about the data science lifecycle, statistical thinking, data quality, reproducibility, interpretability of ML methods, data ethics ...
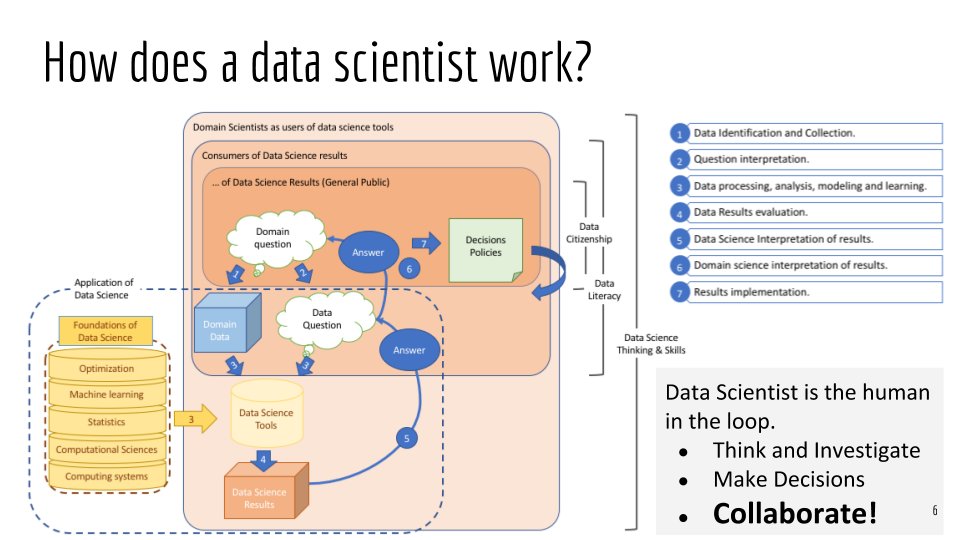
Therefore ...
We created a project-based bootcamp based on @ProPublica's recidivism story https://t.co/Pl88fscGb3. In 5 days, student teams carried out the tasks of map research question, apply DS tools, interpret results, discuss results in the light of the original research questions. 4/9— Tian Zheng (@tz33cu) June 20, 2018
In 5 days, student teams carried out the tasks of
Day 1: studied the news coverage on @ProPublica story, discussed "what is fairness?" (taking notes from @mrtz's fairmlclass.github.io), played the Ultimatum Game, watched how monkey perceives fairness and failed to balance 2 definitions of fairness.
- mapping research question - understand the domain question, identify data for answering the question and interpret the domain question to a data question;
- applying DS tools - for the data question, carry out exploratory data analysis, create data visualization and choose appropriate models and machine learning algorithms;
- interpreting and discussing results - what do the data results suggest in the light of the original research questions.
Here is the approximate schedule of our bootcamp.
Day 2a: teams studied @ProPublica's detailed account of their analysis (propublica.org/article/how-we…). Each team presented a part of the report. One team studied in detail how "recidivism" is defined in the study and commented "whether we should even be predicting recidivism."
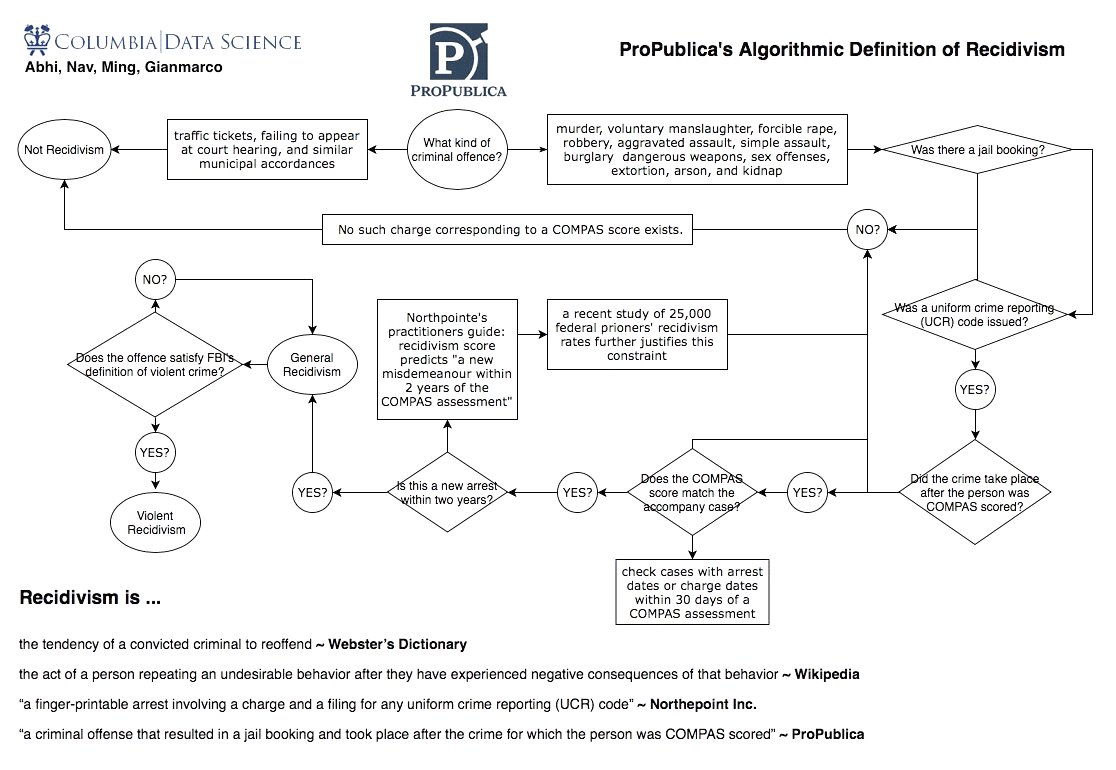
Day2b-Day3a: teams were then tasked to reproduce the analysis using data and codes shared (github.com/propublica/com…) and asked to explore the data more.
Day 3b: we studied research on interpretable ML for recidivism prediction (arxiv.org/abs/1503.07810). We focused on how new ML tools were developed, evaluated and compared with conventional approaches; and how the evidence supporting new research presented and communicated.
Day 4-5: the teams worked on one idea they'd like to try with the data and presented their final projects. We didn't find a solution but we asked many good questions. Read more about our bootcamp at datascience.columbia.edu/scholars/bootc…